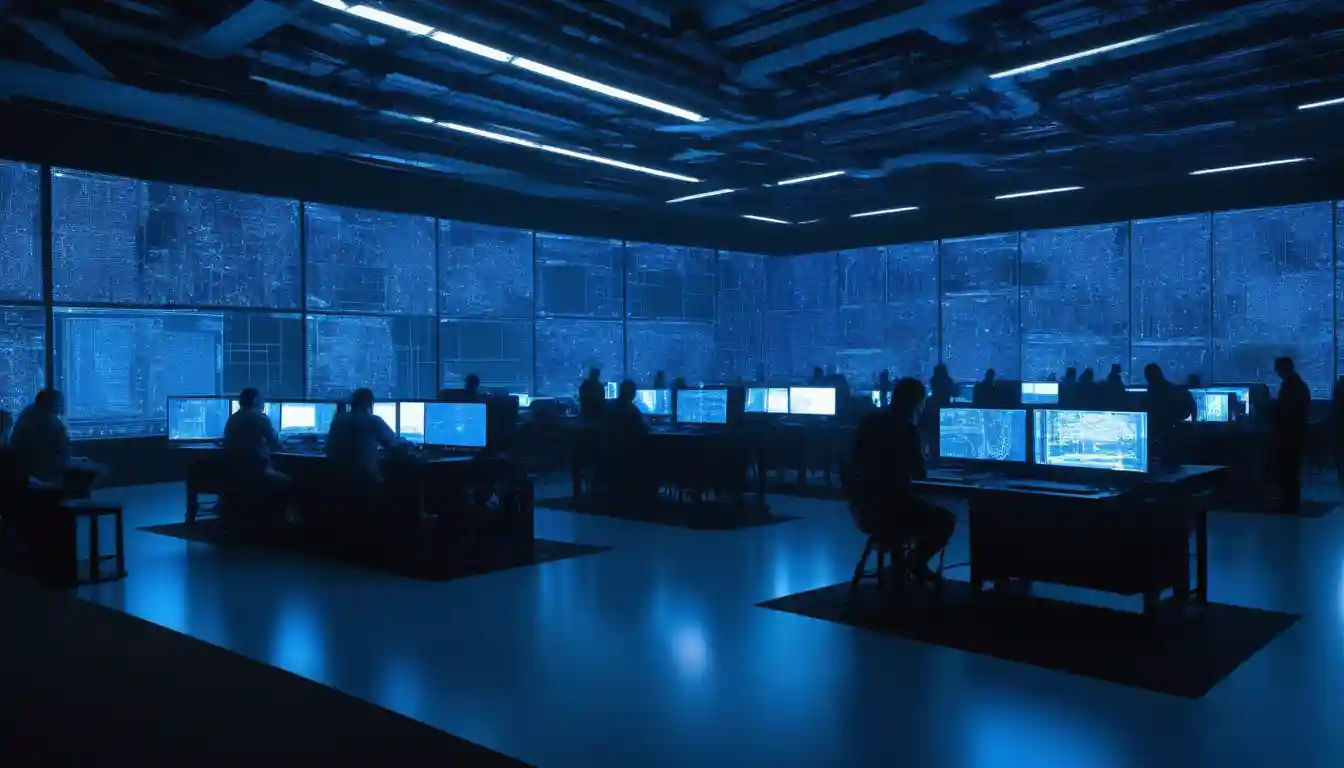
AMD-Backed TensorWave Raises $100 Million to Deploy Massive AI GPU Cluster
AMD's Dark Horse: TensorWave Secures $100 Million to Challenge Nvidia's AI Dominance
LAS VEGAS — In a sun-drenched data center on the outskirts of Las Vegas, rows of gleaming server racks pulse with activity. The heat radiating from thousands of processors is palpable, even through sophisticated cooling systems. This is the nerve center of TensorWave, an upstart that has suddenly emerged as a significant challenger in the intensely competitive AI computing market.
TensorWave announced today it has secured $100 million in Series A funding co-led by Magnetar and AMD Ventures, with participation from Maverick Silicon, Nexus Venture Partners, and new investor Prosperity7. The funding arrives as the company deploys over 8,000 AMD Instinct MI325X GPUs for a dedicated AI training cluster — positioning the firm as potentially the largest AMD-focused AI infrastructure provider in a market overwhelmingly dominated by Nvidia hardware.
"This funding propels TensorWave's mission to democratize access to cutting-edge AI compute," said Darrick Horton, CEO of TensorWave, in the company's announcement. "Our 8,192 Instinct MI325X GPU cluster marks just the beginning as we establish ourselves as the emerging AMD-powered leader in the rapidly expanding AI infrastructure market."
Memory Advantage in a Congested Market
While TensorWave represents a tiny fraction of the overall AI compute landscape compared to giants like CoreWeave and Lambda Labs, its strategic focus on AMD's technology offers a technical advantage that some AI developers find increasingly appealing: memory capacity.
AMD's Instinct MI325X GPUs provide up to 128GB of HBM3 memory per card — double the memory of comparable Nvidia offerings. This additional memory headroom creates a significant edge for training large AI models that frequently strain conventional GPU memory constraints.
"The memory capacity differential is crucial," said a machine learning researcher at a financial services firm. "Many of our models are limited by memory, not raw compute power. Having that extra breathing room makes previously impossible workloads suddenly feasible."
For TensorWave, this technical differentiation comes at a critical moment. The global AI infrastructure market is projected to exceed $400 billion by 2027, according to industry projections. Yet acquisition of suitable AI compute resources remains one of the most significant barriers to AI development and deployment for many organizations.
Scaling Amid Fierce Competition
TensorWave claims to be on track to close the year with a revenue run rate exceeding $100 million — representing a 20-fold year-over-year increase. While impressive for a Series A company, this places TensorWave far behind established competitors. CoreWeave, backed by Nvidia, reported $1.92 billion in 2024 revenue and holds a $23 billion valuation. Lambda Labs, another competitor, saw revenues grow from $70 million in 2021 to approximately $200 million in 2024.
"The $100 million we've secured will transform how enterprises access AI computing resources," said Piotr Tomasik, President of TensorWave. "Through careful cultivation of strategic partnerships and investor relationships, we've positioned TensorWave to solve the critical infrastructure bottleneck facing AI adoption."
However, industry analysts point to significant challenges ahead. Nvidia controls more than 80% of the data center AI chip market, supported by its mature CUDA software ecosystem that many AI developers are reluctant to abandon. AMD's alternative software stack, ROCm, while improving, still lacks the ubiquity and developer familiarity of Nvidia's platform.
"TensorWave isn't just bringing more compute but rather an entirely new class of compute to a capacity-constrained market," said Kenneth Safar, Managing Director at Maverick Silicon. "We think this will be highly beneficial to the AI infrastructure ecosystem writ large."
Price War Looming
The AI infrastructure landscape is increasingly crowded with well-funded competitors. CoreWeave has raised approximately $12.9 billion in debt to scale data centers around Nvidia GPUs. Lambda Labs secured a $500 million asset-backed loan collateralized by Nvidia chips. Meanwhile, major cloud providers like AWS are aggressively pricing their own AI chips, with AWS Trainium reportedly offering cost advantages of 30-40% compared to Nvidia-based solutions.
TensorWave's AMD-focused strategy may provide cost advantages, with channel checks suggesting AMD silicon is approximately 20% cheaper per floating-point operation than comparable Nvidia offerings. This efficiency could allow TensorWave to undercut competitors on price while maintaining healthy margins, particularly for memory-intensive workloads.
"Memory bottlenecks are the hidden constraint in many production AI systems," noted an industry consultant who specializes in AI infrastructure optimization. "The cost per training run isn't just about raw teraflops anymore — it's about whether you can fit your model in memory efficiently."
Supply Chain Resilience
One potential advantage in TensorWave's AMD partnership lies in chip supply availability. While Bain warns of a 30% chip shortfall through 2026, AMD's strategic investment suggests TensorWave may have privileged access to hardware that remains in short supply.
"AMD's strategic investment in TensorWave reinforces the commitment of AMD to expand its footprint in the AI infrastructure space," said Mathew Hein, SVP Chief Strategy Officer & Corporate Development at AMD.
This partnership could prove vital as global demand for AI compute continues to outstrip available supply, particularly as enterprises seek alternatives to heavily subscribed Nvidia-based infrastructure.
The Road Ahead
TensorWave faces formidable obstacles despite its promising start. The company's reported revenue run rate likely depends on a small number of large customers, creating potential concentration risk. Additionally, building and maintaining data centers at scale requires massive capital investment — the current 8,000 GPU deployment likely represents an investment of hundreds of millions of dollars in hardware alone.
The company will need to demonstrate that it can attract mainstream AI developers who have built their workflows around Nvidia's ecosystem. This migration challenge has proven difficult for previous AMD-focused initiatives in the AI space.
"The biggest barrier isn't hardware performance — it's software inertia," explained a veteran of several AI infrastructure startups. "Developers have years of work invested in CUDA-optimized code bases. Even with superior hardware specs, convincing them to port their workloads is an uphill battle."
For now, TensorWave's success appears contingent on three critical factors: the maturation speed of AMD's software ecosystem relative to Nvidia's entrenched position, pricing dynamics in an increasingly competitive market, and the company's ability to secure the additional capital necessary to scale beyond its initial deployment.
As global AI compute demand continues its explosive growth, TensorWave represents an intriguing alternative in a market yearning for options beyond the established players. Whether it can transform its technological differentiation into sustainable business advantage remains to be seen.