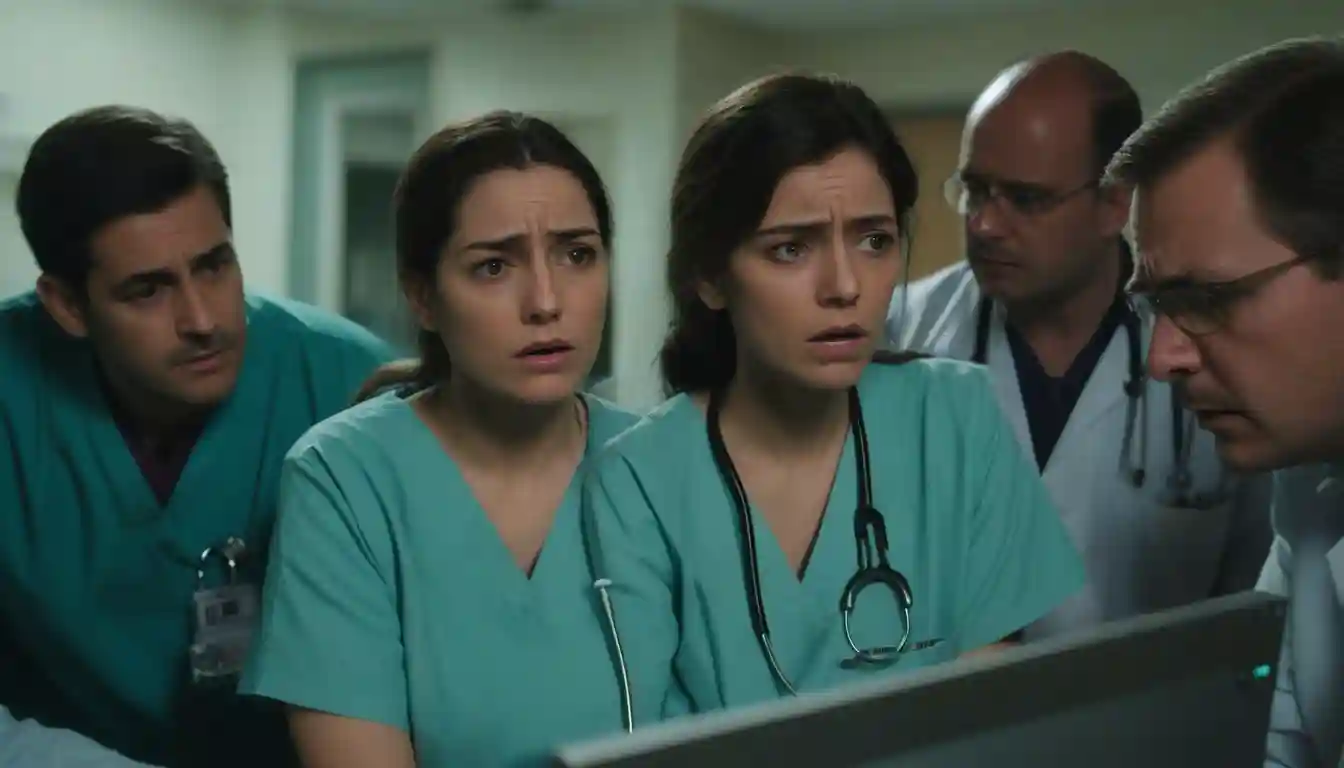
Medical AI Under Scrutiny: Top LLM Flop at Diagnosis, Outperformed by Random Guesses
Researchers found LLMs perform worse than random guesses on medical questions
A recent study by researchers from the University of California, Santa Cruz, and Carnegie Mellon University has raised concerns about the reliability of Large Multimodal Models (LMMs) in the medical field. The research, titled "Worse than Random? An Embarrassingly Simple Probing Evaluation of Large Multimodal Models in Medical VQA," found that state-of-the-art LMMs, such as GPT-4V and Gemini Pro, performed worse than random guessing on medical diagnosis questions. The study introduces the Probing Evaluation for Medical Diagnosis (ProbMed) dataset to assess LMM performance in medical imaging through probing evaluation and procedural diagnosis, highlighting significant limitations in the current models' ability to handle fine-grained medical inquiries.
Key Takeaways
- Performance of LMMs: Top-performing models like GPT-4V and Gemini Pro were found to perform worse than random guessing on specialized diagnostic questions.
- ProbMed Dataset: A new dataset was introduced to rigorously evaluate LMM performance in medical imaging through probing evaluation and procedural diagnosis.
- Adversarial Pairs: The study used adversarial pairs in the evaluation process to test the models' robustness and reliability, revealing a significant drop in accuracy when these pairs were introduced.
- Domain-Specific Knowledge: Models like CheXagent, trained on specific modalities, demonstrated the transferability of expertise across different modalities of the same organ, emphasizing the importance of specialized domain knowledge.
Deep Analysis
The study conducted a systematic evaluation using the ProbMed dataset on seven state-of-the-art LMMs to identify their strengths and weaknesses in real-life imaging diagnostics. The evaluation included both general and specialized models, focusing on their ability to answer questions related to medical imaging.
The introduction of adversarial pairs, which are question-answer pairs designed to challenge the model's ability to validate the absence of certain characteristics, had a significant impact on model performance. The accuracy of some models dropped drastically, with an average decrease of 42.7% across the tested models when adversarial pairs were added to the VQA-RAD dataset, and an average decrease of 44.7% in ProbMed.
The study also revealed that even the most robust models experienced a minimum drop of 10.52% in accuracy when tested with ProbMed's challenging questions. This highlights the critical role of probing evaluation in comprehensively evaluating Med-VQA performance.
Impact on Public Confidence and Funding
The findings of the study not only have technical implications but also broader societal and economic consequences. Here are some additional considerations on the impact:
-
Public Confidence in Medical AI: The revelation that advanced LMMs perform worse than random guessing on certain medical questions could undermine public confidence in the effectiveness and safety of AI-driven medical tools. Trust is a critical component in healthcare, and patients are more likely to adopt and benefit from AI technologies if they believe these systems are reliable and accurate.
-
Impact on Funding and Investment: The medical AI industry relies heavily on investment to fuel research and development. Negative findings like these could lead to reduced investor confidence, resulting in less funding for startups and established companies alike. This could slow down the pace of innovation and the development of potentially life-saving technologies.
-
Regulatory Implications: As concerns about the reliability of LMMs in medical diagnosis grow, there may be increased pressure on regulatory bodies to impose stricter guidelines and oversight. This could lead to a more cautious approach to approving new AI technologies in healthcare, potentially delaying their availability to patients.
-
Ethical Considerations: The ethical use of AI in healthcare is paramount. If LMMs are found to be unreliable, it raises questions about the ethical responsibility of developers and healthcare providers to ensure that AI systems are thoroughly tested and validated before being used in clinical settings.
-
Patient Safety and Outcomes: Ultimately, the most significant impact is on patient safety and health outcomes. If medical AI systems are not reliable, there is a risk that they could provide incorrect information or diagnoses, potentially leading to inappropriate treatment or delayed care, which could have serious consequences for patients.
-
Market Dynamics: The study's findings could also affect the competitive landscape of the medical AI market. Companies with robust, well-validated products may gain a competitive edge, while those with less reliable offerings may struggle to maintain their market position.
-
Research Priorities: The results may prompt a shift in research priorities, with more focus on developing and validating robust evaluation methodologies and on integrating domain-specific expertise into AI models to improve their reliability and performance in medical applications.
In light of these potential impacts, it is crucial for the medical AI community to address these concerns transparently and proactively. Open communication about the current limitations of AI technologies, coupled with a commitment to ongoing improvement and validation, will be key to maintaining public trust and securing the future of AI in healthcare.
Did You Know?
- Transferability of Expertise: The study found that specialized knowledge gained on chest X-rays can be transferred to other imaging modalities of the same organ in a zero-shot manner, indicating the potential for cross-modality expertise transfer in real-life medical imaging diagnostics.
- Importance of Robust Evaluation: The research underscores the urgent need for more robust evaluation to ensure the reliability of LMMs in critical fields like medical diagnosis.
- Potential Impact on Healthcare: The findings of this study have broader implications for improving diagnostic accuracy and patient care, but also highlight the risks of deploying unreliable models in healthcare.
In conclusion, the study emphasizes the need for rigorous testing, continuous performance monitoring, and the incorporation of domain-specific expertise to enhance the development of trustworthy AI systems in healthcare and ultimately improve patient outcomes.